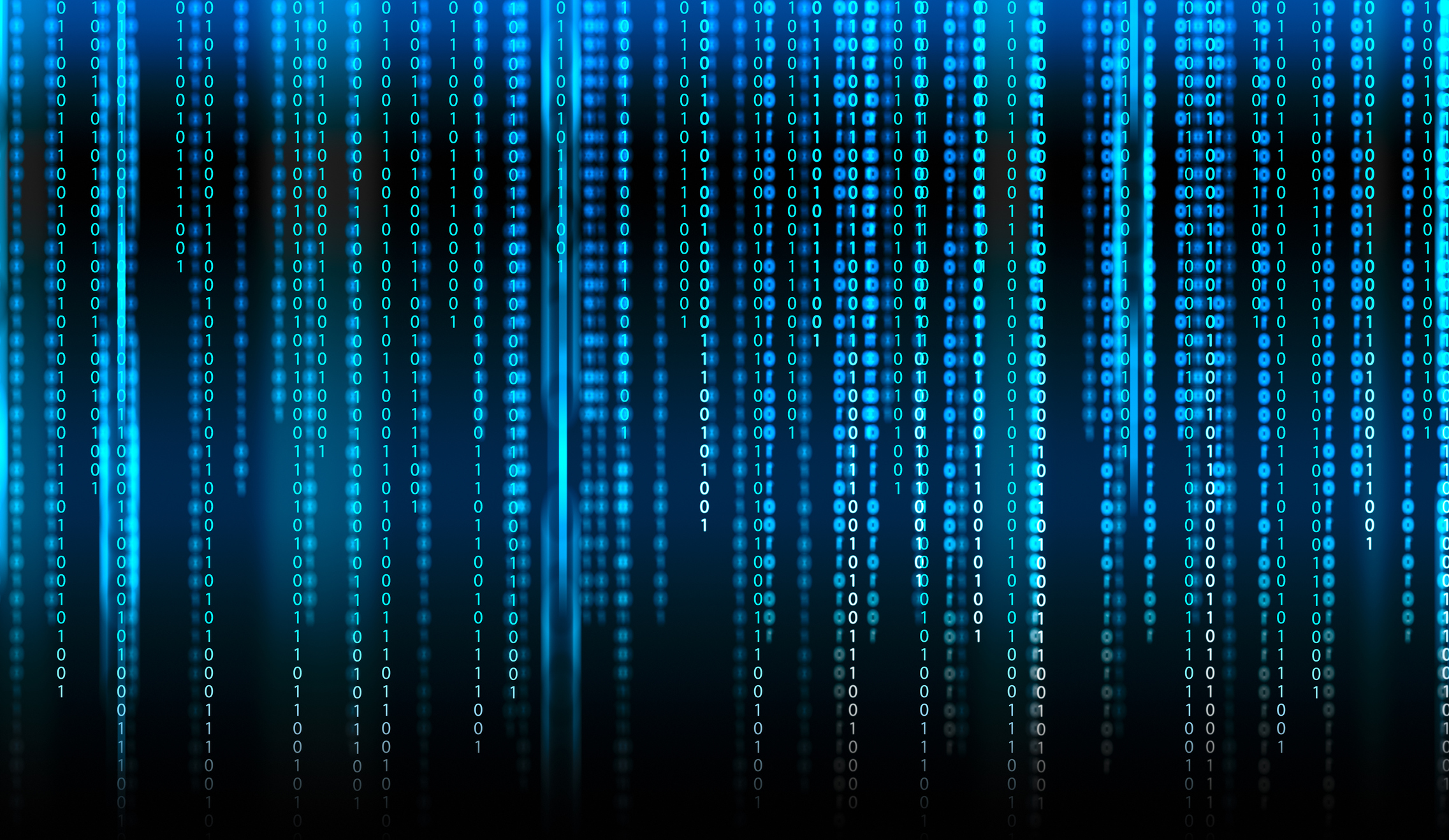
The first movie I saw in a movie theater was Star Wars. I was five and couldn’t hold the seat down. My favorite book series as a pre-teen was Madeleine L'Engle’s Time Quintet. I took extra science classes in high school and I might have been the only person in my Science, Technology, and Ethics class in college that actually enjoyed the required readings. I dressed up as Trinity from The Matrix more than once. I have been thinking about the potential benefits and consequences of machine learning outpacing human intelligence and the concept Singularity for quite some time. So, I wasn’t surprised — and had actually been expecting — a call to come to us at NSVRC about using artificial intelligence (AI) to interrupt sexual assault. What was surprising was that it came during a global pandemic.
The pandemic has been hard on our brains, but we have been trying to figure out how technology can be used to prevent violence for a really long time. There have been a lot of missteps along the way and some recent successes when partnering with technology companies. While I’m not going to go into all my thoughts about AI in this blog post, I am going to recommend three things related to data and technology that those of us working to end sexual harassment, abuse, and assault may consider weaving into our work. While it has been almost a year since the documentary Coded Bias premiered at the Sundance Film Festival, this post is wholly inspired by it and the work of MIT Media Lab researcher and Algorithmic Justice League (AJF) Founder, Joy Buolamwini.
The film examines the harm caused by racial, gender, and class bias that is embedded into AI and machine learning. This harm impacts all facets of human existence, from housing and health, to employment and education, and ultimately to our freedom. If you don’t see the connections to sexual violence right away, check out NSVRC’s information on risk and protective factors.
Here are three things that prevention educators and program evaluators can learn from the Algorithmic Justice League:
1. Examine how data is produced.
NPR’s Hansi Lo Wang talked about how data is “produced” and “not just waiting out there in the wild” in relation to the U.S. Census. This framework is important to help us examine bias in our national data sets as we evaluate our own sexual violence prevention programs.
2. Plan how you will prioritize data equity, continuously.
Preventing sexual assault, harassment, and abuse requires that we stop abuses of power that contribute to inequalities. Since we are producing, sharing, and using data everyday in our field, we need to have critical conversations about what story our communities need to tell and how data can empower them to tell it. Listen to our podcast with Heather Krause from We All Count and check out their seven-stage Data Equity Framework.
For the evaluators out there, you might want to take a look at this new three-part series of guides from the W.K. Kellogg Foundation on how to incorporate racial equity as a core value in designing and implementing evaluation activities. If you are looking for some help in how to talk within your organization or community about the connections between sexual violence and oppression, check out NSVRC’s infographic.
3. Amplify movements working for justice.
To create a world that is safe, healthy, and just, we need to work in solidarity with other movements for change. You can join the movement for equitable and accountable AI by joining the Algorithmic Justice League. If AI isn’t showing up in your daily life like it is mine, you can also look for additional inspiration from the 2021 National Sexual Assault Conference workshop, Social Justice Movements Working in Solidarity.